Within a machine learning workflow, it begins with the manual extraction of the most relevant aspects of the images, but with a deep learning workflow , these relevant aspects are extracted directly from these images.
Deep learning is about complete learning, that is, the data is unprocessed and the machine must learn on its own or automatically.
Deep learning algorithms scale with data, but shallow learning requires gambling email list convergence. Convergence indicates that machine learning methods can reach a plateau at a certain performance level , such as when adding more training data to the neural network.
[Tweet “Deep learning is about complete learning, that is, the data is raw and the machine must learn automatically.”]
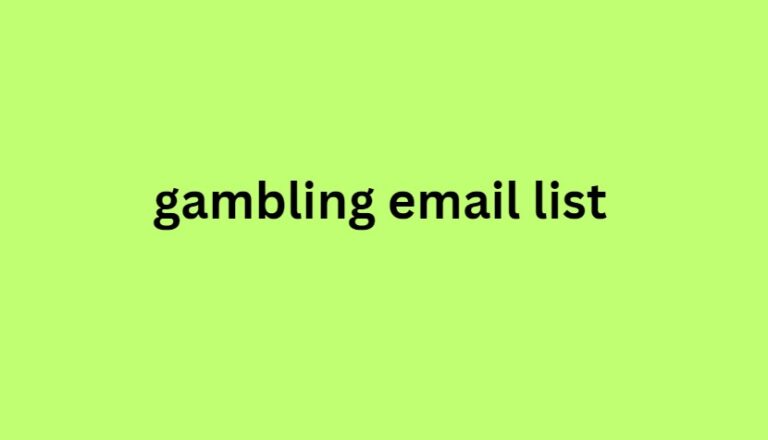
All machines that use algorithms work with neural networks, but deep learning goes further and works with “deep neural networks.”
This is due to the number of hidden or concealed layers with which this technological model operates.
This number has been estimated at more than one hundred and fifty layers, and that is where its complexity in operation lies.
The way deep learning works is by training models using data sets that are labeled and a dynamic in the neural network in which they learn from the data without the manual requirement to achieve this.
On the other hand, one of the most popular types of deep neural networks are those called convolutional neural networks (CNN or ConvNet).
The way it works is that a CNN convolves the learned aspects with the input information and uses 2D convolutional layers, which makes this dynamic conducive to processing 2D data such as images.
These CNNs, on the other hand, eliminate the need for manual feature extraction, so the identification of the aspects used in the classification of the 2D material is required.
Therefore, CNN extracts the most relevant features directly from the images.
These features have not been trained before, since they are learned while the network is trained with a collection of images.
This makes the deep learning model as precise or specific to missions like computer vision, as it is to classify things.
In conclusion, CNNs “learn” to know what the different features of an image are through many hidden layers, where each of them increases the complexity of the learned object features.
To help you understand better, we give you an example: the first hidden layer learns about colors, while the second learns how to recognize the edges of images.